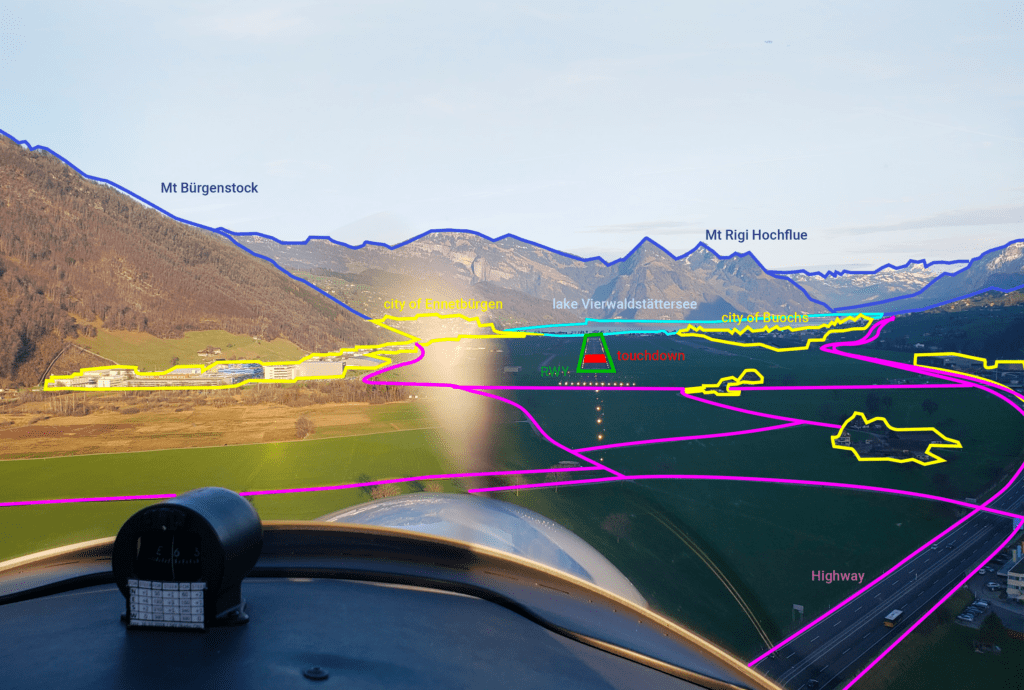
The visual positioning system (VPS) runs Daedalean’s algorithms to report to flight control equipment or to a human pilot the data on position, altitude, heading, ground and vertical speed, accelerations (and corresponding uncertainties) and gives landing guidance. (Daedalean)
Technologies like artificial intelligence (AI) and machine learning (ML) continue to be a black box for many in the aviation industry, Luuk van Dijk, founder and CEO of Daedalean, said during a Revolution.Aero Town Hall. However, van Dijk sees AI/ML as a way to simplify aviation operations and improve on current human piloting capabilities all while being safety-critical certified.
“It was delightful to see a lot of misunderstanding and preconceptions about how impossible this [AI] was,” van Dijk said. “…Our goal is to develop the kind of machine equivalent of human capability, which would call AI so that we can get to this ultimate form of autonomy.”
Van Dijk saw a gap in the developing electric vertical take-off and landing (eVTOL) industry four years ago and decided to tackle it, he said during the webinar. While others in the industry were building new energy storage and propulsion systems, he would tackle AI, which he believed others lacked the skill set and risk appetite for.
“We looked at machine learning systems, and everybody knows these are black boxes and you can never get them certified because nobody knows how they work,” van Dijk said. “So I figured while everybody thinks that, we can start working on understanding how they work and not only build the systems that are good enough to make the kind of judgment calls that the human makes today, but also build the tools and the instruments and the mode of thinking for a regulator like EASA [European Union Aviation Safety Agency] and the FAA [Federal Aviation Administration] to see that these things are fit for purpose and safe.”
On Apr. 1, Daedalean and EASA jointly published a report entitled, “Concepts of Design Assurance for Neural Networks.”
The report is the result of 10 months of work between EASA and Daedalean. According to EASA, the jointly published report is the result of a 10-month collaborative project between the two organizations with the goal of investigating the challenges and concerns of using Neural Networks (NN) in aviation.
AI technology has come a long way, starting with playing chess and then recognizing cats in YouTube videos, van Dijk said. Now that computational power and big data are on the scene, AI is ready to advance to bigger tasks, including safety critical flight controls and navigation systems.
Before AI is ready to pilot an aircraft, there has to be a redefinition of the mystery behind how it works. Van Dijk said he prefers to talk about machine learning systems to get rid of the idea that AI is a “magic black box.”
“You take well-defined subtasks of the art of flying and then you can build a system that meets the VFR [visual flight rules] requirements of seeing other aircraft on visual, even the ones that don’t show up on a radar, or ones that can recognize the runway on visual and make the call to abort or not because there’s the burning wreck of the previous guy to try to land there,” van Dijk said. “There are clearly there’s no equivalent system today and you have to solve these problems.”
Van Dijk admits that the stakes are high for this technology. The first accident will have massive repercussions for the industry, van Dijk said. This is why he says getting a pass for developing a non-deterministic system would be a big mistake.
AI will have to prove it is safer than human piloting, van Dijk said. These systems will have to deal with uncertainty in real-world environments. The avionics industry deals with uncertainty by outlawing it and passing it to the human pilot. While uncertainty is a problem with AI piloted systems, it is also a problem in the environment for human pilots.
“The trick is that worrying about the non-determinism is a bit of a red herring because the system itself is perfectly reproducible and analyzable,” van Dijk said. “What you have to do is you have to show that the data that you trained and tested on in the lab is sufficiently representative of the randomness you’re going to find out in the real world.”
While certification of AI will not be trivial or easy, van Dijk said, it is not impossible. He compares the path for AI certification to how avionics software is certified by the FAA, DO-178C.
In August, Daedalean confirmed a partnership with business and general aviation aircraft avionics maker Avidyne to develop what they describe as the first ever machine learning-based avionics system. It is designed as several cameras and a powerful computation unit, interfacing to other aircraft electronics, capable of detecting any airborne or ground-based hazard.
“Nothing in the process actually guarantees that your system will then fail not more than once in 107 hours of flight,” van Dijk said. “And what you have to realize is that’s already an exception, compared to all the other systems in your aircraft.”
It is not a perfect system, van Dijk admits, but the risk is manageable. However, this does not mean that regulators should let up on the pressure to make these systems as safe as possible because this will allow more public acceptance of AI piloting aircraft.
“The paradox is, and this is actually kind of surprising that by going for this harder class of systems, we can actually get to a higher level of safety, provided we do it right,” van Dijk said. “And then the other thing I think is important is that we, and the public, should not be satisfied until we have actually proven that, so that’s actually a very important point. We should not have the attitude that you know the old regulator should step aside and let the modern age take over.”