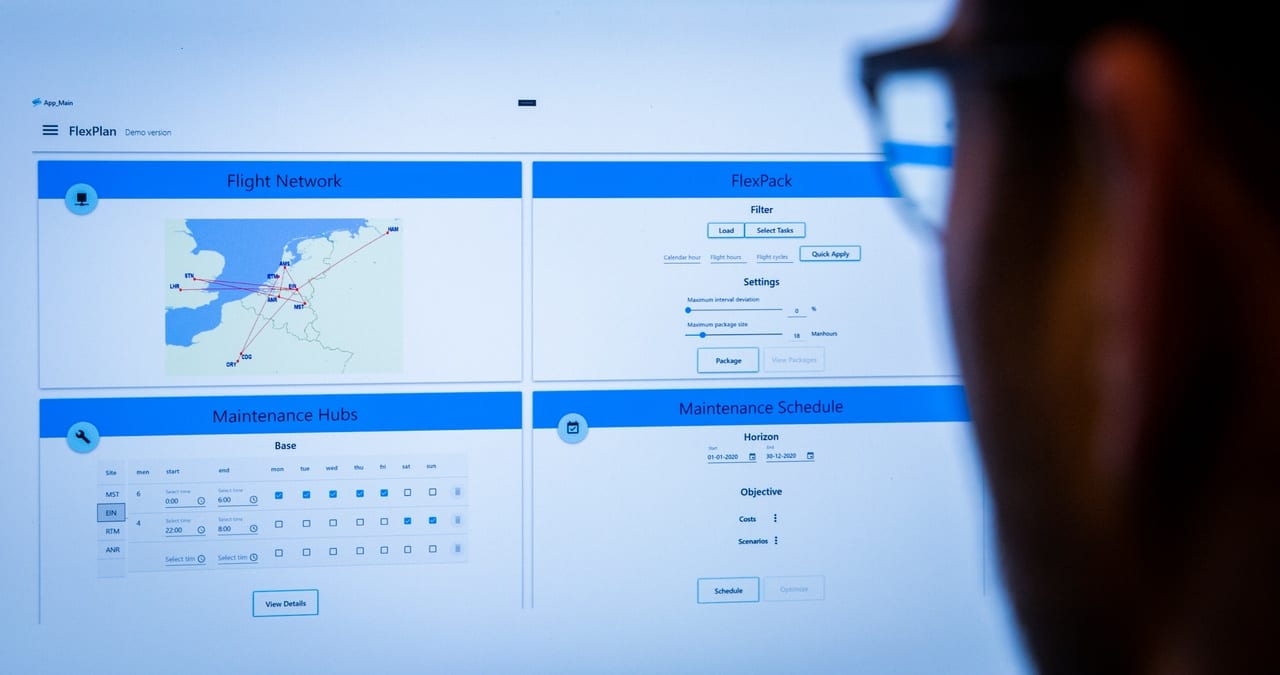
Amsterdam-based applied science institute Netherlands Aerospace Laboratory (NLR) is in discussion with several airlines about the ongoing development of its artificial intelligence algorithms, FlexPlan, designed to solve what they describe as an aircraft maintenance “subproblem” of preventive maintenance planning.
As a European Union Aviation Safety Agency (EASA) qualified entity in product certification and flight standards approval, the institute is involved in a range of other research projects and initiatives as well. In February, NLR and Delft University of Technology published a white paper presenting their joint vision on specific technologies and research and development activities Dutch research institutes and companies should engage in to help achieve a climate neutral European air transport system by 2050.
Their Air Traffic Control Research Simulator (NARSIM) team is also involved in ongoing research and development of prototype air traffic software, testing new safety features and creating new designs for on-screen radar displays among their other R&D activities.
FlexPlan is their first major research projects focused on using artificial intelligence for aircraft maintenance. The algorithm currently being tested for FlexPlan has the ability to read a maintenance planning document, interpret the applicability of each individual component or system driven task in the document and then automatically generate an aircraft maintenance program aligned to a specific airline’s flight schedule, fleet type and route structure.
A key capability within the algorithm is its ability to update maintenance slots if last-minute changes to a flight schedule occur.
“It is our task to be ahead of the market and develop solutions that fulfill current and future demand of the aerospace industry,” Dr. Arjan de Jong, program manager for maintenance engineering, management and technology at NLR told Aviation Today. “For preventive maintenance planning, we have developed a series of algorithms that each solve a sub-problem of preventive maintenance planning. The optimization flow is able to efficiently evaluate many options and construct efficient (base) maintenance schedules for a fleet of aircraft. We implemented the algorithms in a generic demonstrable tool.”
A growing number of civil and military aircraft operators have been collaborating with aviation OEMs and IT industry suppliers of applications and algorithms that can integrate the use of artificial intelligence and machine learning into their aircraft maintenance processes.
What has driven the growth of these initiatives is the expanding availability of app store style interfaces that give airlines an easier bridge between the data generated by their airplanes daily and the machine learning models and algorithms that can take that data and turn it into actionable insights for maintenance technicians such as work orders or parts replacement needs.
Companies on the aviation and IT side of the supply of these algorithms and applications are competing, partnering and constantly developing new insights and understandings of where artificial intelligence and machine learning algorithms for aircraft maintenance make sense.
“To bring the product into production and integrate it into a real-life environment we are constantly cooperating with, and looking for partners, since each airline has specific operational constraints and business logic that need to be addressed during implementation,” de Jong said.
That transition of FlexPlan from the development to operational phase will require more partnerships with airlines, maintenance organizations, and “software houses” according to de Jong. Their R&D with the algorithm is conducted based on available data-sets including maintenance planning documents, flight schedules, aircraft configurations and the location of maintenance hubs.
“FlexPlan divides the scheduling problem into multiple sub-problems and uses a unique technique to solve/optimize each of these sub-problems. Some of these techniques include Unsupervised Learning (UL), Mixed Integer Linear Programming (MILP), Dynamic Programming (DP), and self-developed heuristics. The interface is simplified for the user to the point where the user only needs to specify its fleet details, task data, flight timetable, and available maintenance resources. The user does not need to specify algorithmic parameters, the optimization flow between the various solvers is automated,” de Jong said.
Several other partnerships between aviation industry companies and IT solutions providers are also slowly making AI applications available to airline maintenance teams and OEMs through automated methods where machine learning models are made available to them through a web interface that allows them to customize the type of data that is ingested by the model, what it learns and what actionable insights it should be generating regularly.
Lufthansa Technik, the maintenance, repair, and overhaul (MRO) division of Lufthansa Group, has worked with data engineering and analytics provider Cloudera to develop a predictive maintenance platform that services a fleet of 5,000 aircraft throughout its global network of 800 MRO facilities. The Cloudera Data Platform, released last year, builds predictive maintenance models based on sets of data that can train machine learning models to determine when a particular aircraft component is approaching failure.
According to an Oct. 14 blog post published by two Cloudera engineers, training data sets allow machine learning models to develop predictions about when engine components are approaching failure. In one example, the Cloudera engineers show how by taking data sets from Kaggle, a site that provides simulation data sets from NASA to measures engine component degradation for turbofan jet engines, integrating different engine parameters into the models based on inputting various engine parameters showing typical sensor values of engine temperature, fuel consumption, vibration, or fuel to oxygen mixture, the models can predict engine failures.
“Each set of training data shows the engine parameters per flight while each engine is ‘flown’ until an engine component signals failure. This is done at both sea level and all flight conditions. This data will be used to train the model that can predict how many flights a given engine has until failure,” the Cloudera blog post says.
Some cloud computing providers and aviation OEMs are also starting down the path of AI and ML development for aircraft maintenance on the military side of the aviation industry. Bell Flight is working with IBM’s Global Business Services to change the way the U.S. Army uses aircraft sensor data to maximize fleet readiness. Bell’s V-280 is one of the companies that was selected by the Army last year to Future Long-Range Assault Aircraft program to find a Black Hawk replacement.
An August 2020 blog post published to IBM’s website by Peter Shultz, head of Bell’s future vertical lift digital systems division, outlined how the helicopter manufacturer is working with IBM to develop AI technologies like deep learning that can find and predict aircraft parts and components that are on the verge of failure.
“The core of our vision is that artificial intelligence technologies like deep learning can find—in fact predict—those risks before they affect performance and combat readiness,” Shultz writes.
As real-time health monitoring of aircraft parts and components becomes more widespread among airlines, the type of technology supplied by NLR has the potential to optimize maintenance planning by improving on-wing or on-aircraft maintenance to reduce disruption. Most aircraft maintenance planning schedules today are developed based on data or anomalies already captured by the system, whereas their algorithm would regularly update the maintenance plan based on changes to the flight schedule.
“NLR has conducted studies for predictive and diagnostic maintenance using explainable AI. For a large fleet of high-performance jets, we determined actual wing load factors. With that information, we could reduce drastically on corrective maintenance,” de Jong said. “We envision data-driven solutions to play a major role in aircraft maintenance in the future. With regards to FlexPlan we have designed and are currently testing additional optimization modules which add more details, business logic, and operational constraints when constructing solutions.”