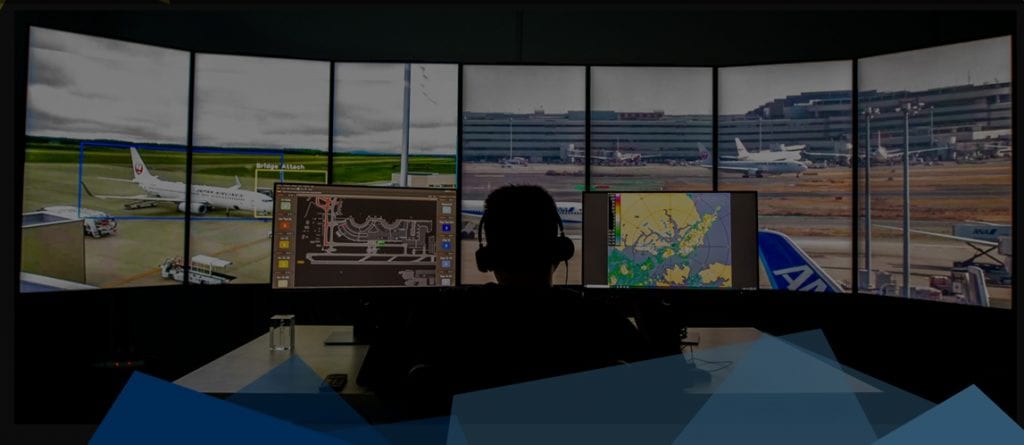
Research on the use of artificial intelligence and machine learning in air traffic management (ATM) has expanded in recent years, but the majority of that research separates humans and machines, a theme among the ATM research community that needs to change, according to a presentation given by Dr. Sameer Alam, Deputy Director of the ATM Research Institute at NTU Singapore.
Alam, whose presentation came during the March 23 “A FLY AI” webinar hosted by the SESAR JU, also leads digital technology developments at NTU Singapore’s $13 million joint research center, the Saab-NTU Joint Lab launched last year in collaboration with Swedish aerospace and defense supplier Saab. His research has centered around the establishment of an AI-Human hybrid model that allows for machine learning techniques to develop intelligent agents capable of augmenting and eventually performing actions in real-time such as conflict resolution, taxi routing, or metering among other controller decision-making processes.
“For AI to play a role in the future of the ATM system, it is very important that we understand and recognize the signal in the noise so we can see what’re the world-changing developments versus the over-hyped press releases,” Alam said.
“There is a need of coming up with solutions that are human and machine co-developed and co- teamed,” he said.

Machine learning models being developed by Alam and NTU Singapore’s ATM research lab focus on AI-Human hybrid concepts. (NTU Singapore)
Among the examples presented by Alam included a classical conflict detection and resolution (CD&R) scenario versus the same scenario using a machine learning model. The demonstration showed how the classical CD&R model uses established horizontal and vertical separation standards and determines whether a conflict is about to occur based on available surveillance data about aircraft flight paths, routes, and airspace positioning.
The machine learning hybrid human-AI model developed by Alam’s team uses the same concept but allows the machine to learn the separation rules used by controllers and the necessary actions to take based on the controller actions observed by the machine over a specific period of time.
“How the controller solved them is what is input into the machine learning models, and this paradigm helps us to learn the rules. Instead of hard coding the rules by getting smart programmers and hard coding the rules, let the machine discover the rule by itself,” Alam said.
“And when the machine is exposed to the unseen conflict scenario, it is expected that it will show these various actions it will take to the controller and the controller provides feedback, about these conflict resolution strategies selected by the machine learning. This is the co-teaming or hybrid human paradigm we’re working on at the moment,” he added.

Alam’s AI-Human air traffic controller hybrid approach explained.
Using airside infrastructure and ground movement radar data at Changi Airport, Alam’s research team has also developed spatial-temporal models of aircraft arrivals and departures at various taxiway intersections to compute conflict probability. These identified intersections are then visualized within an airport diagram as hotspots that controllers need to have heightened awareness of.
One other project in development by Alam involves the use of a machine learning algorithm that provides a confidence meter for clearing aircraft for departures based on wake vortices of arrivals.
“We’re looking for learning fleet departures, learn arrival stream, learn wake vortex, learn [runway occupancy time] ROT, and be able to predict the inter-arrival gaps and push the departures with an indicated confidence level,” Alam said.
Overall, the central hybrid AI-Human model envisioned by Alam and the NTU Singapore researchers involves the extraction of human actions as intelligence to feed machine learning algorithms from large swaths of data about the actions taken by controllers in different decision-making scenarios.
By converting this extracted data into patterns and generalizations, the machines or AI agents can use the patterns to predict the action they should take, and take that action. With a human in the loop, those patterns are constantly updated based on human action.
When asked during the webinar what strategy his team is taking to avoid the development of intelligent agents that learn bad air traffic controller behavior, Alam explained how his team is approaching that challenge.
“There is a need to de-bias the data, and machine learning techniques allow us to amplify the noise or the outliers that can be identified. There’s a need to establish an envelope or set of boundaries that we see in the data, these are the techniques of de-biasing the data and learning normal behavior from the data,” Alam said. “As more data becomes available, the law of averages will kick in and we will be able to see normalized behavior.”